Share
Tweet
Share
Share
The integration of artificial intelligence (AI) into healthcare has opened new frontiers for improving patient care and operational efficiency. Among its most promising applications is predictive analytics, which uses historical data, machine learning (ML), and advanced statistical methods to forecast future trends, risks, and outcomes. This transformative technology is reshaping healthcare by enabling earlier interventions, optimizing treatment, cutting costs, and revolutionizing patient monitoring. This article provides a detailed exploration of how AI-driven predictive analytics is addressing critical challenges in healthcare, with a particular focus on improving outcomes, reducing costs, and advancing patient monitoring.
Understanding AI-Driven Predictive Analytics
Predictive analytics in healthcare leverages vast datasets, including electronic health records (EHRs), medical imaging, genomic data, and even external environmental and social data, to forecast future health outcomes. AI and machine learning enhance this process by automating the analysis, identifying complex patterns, and providing actionable insights faster and more accurately than traditional methods.
By adapting to new data, these AI systems improve their predictions over time. For example, they can forecast disease outbreaks, predict patient readmissions, or suggest personalized treatment plans. This adaptability and precision make AI-powered predictive analytics a game-changer in both clinical and operational settings.
Enhancing Healthcare Outcomes
- Early Disease Detection and Diagnosis
AI-driven predictive analytics enables early detection of diseases such as cancer, diabetes, and cardiovascular conditions. For instance, ML models trained on imaging datasets can identify subtle anomalies in radiology scans, such as tumors or plaque buildup in arteries, with a higher degree of accuracy than human radiologists.
Furthermore, predictive analytics can assess genetic predispositions and lifestyle factors to identify patients at risk for chronic diseases. By detecting these risks early, healthcare providers can initiate preventive measures, improving patient outcomes and reducing the long-term burden of disease.
- Personalized Medicine
Predictive analytics is the backbone of precision medicine, which tailors treatment plans to individual patient needs. AI algorithms analyze a combination of genetic, environmental, and clinical data to predict the effectiveness of specific treatments.
For instance, in oncology, AI can identify genetic markers in a patient’s tumor to recommend targeted therapies. In pharmacology, predictive models can forecast adverse drug reactions based on a patient’s genetic profile, helping clinicians prescribe medications with greater confidence and fewer side effects.
- Reducing Complications and Readmissions
Hospitals face significant penalties for high readmission rates, and predictive analytics offers a solution. By analyzing patient data, AI can identify individuals at high risk of complications or readmissions after discharge. Healthcare providers can then design tailored follow-up plans, including regular check-ins, remote monitoring, or lifestyle recommendations.
For example, AI tools can predict exacerbations in chronic obstructive pulmonary disease (COPD) or heart failure, prompting early interventions that reduce the likelihood of hospital readmissions.
- Improved Management of Chronic Conditions
Chronic diseases account for the majority of healthcare spending globally, and managing these conditions effectively is a priority. Predictive analytics supports chronic disease management by forecasting disease progression and enabling timely interventions.
In diabetes care, for example, wearable devices and AI algorithms can continuously monitor blood sugar levels and predict potential spikes or drops. Patients receive real-time alerts to adjust their diet, insulin dosage, or physical activity, preventing complications and enhancing quality of life.
Driving Cost Reduction
- Optimizing Resource Allocation
AI-powered predictive analytics helps healthcare providers allocate resources efficiently by forecasting patient volumes, identifying high-risk cases, and anticipating demand for specific services. For example, during flu season, predictive models can estimate the number of patients requiring vaccination or intensive care, allowing hospitals to plan staffing and supply chain logistics effectively.
- Preventing Medical Errors
Medical errors are a significant contributor to healthcare costs and patient harm. AI systems can analyze patient data, including medication history and lab results, to flag potential errors, such as incorrect drug dosages or harmful drug interactions. By reducing errors, healthcare providers can avoid costly corrective treatments and improve patient safety.
- Reducing Emergency Room Visits
Emergency room (ER) visits are costly, often arising from preventable conditions. Predictive analytics can identify patients at risk of acute episodes, such as asthma attacks or cardiac events, and provide early warnings. For instance, AI-powered wearable devices monitor vital signs and alert patients to seek care before their condition worsens, reducing unnecessary ER visits.
- Detecting Fraud and Waste
Fraudulent claims and billing errors inflate healthcare costs. AI algorithms trained to analyze claims data can identify anomalies, such as duplicate charges or suspicious billing patterns, enabling insurers to detect and address fraud proactively.
Advancing Patient Monitoring
- Wearable Technology Integration
Wearable devices, such as smartwatches and biosensors, continuously collect health data, including heart rate, blood pressure, oxygen levels, and activity patterns. Predictive analytics processes this data to detect abnormalities, such as arrhythmias or oxygen desaturation, in real time.
For example, a wearable ECG device can predict atrial fibrillation before symptoms manifest, enabling early treatment and reducing the risk of stroke. Similarly, fitness trackers combined with AI can help patients manage chronic conditions like obesity or hypertension by providing personalized activity and dietary recommendations.
- Remote Patient Monitoring (RPM)
RPM has become a cornerstone of modern healthcare, particularly for managing chronic diseases and post-surgical recovery. AI-powered RPM systems use predictive analytics to track patient data remotely and forecast potential complications.
For example, in heart failure patients, AI algorithms can analyze data from weight scales, blood pressure monitors, and symptom logs to predict fluid retention or worsening conditions, prompting timely interventions. This reduces the need for hospital visits while ensuring continuous care.
- Real-Time ICU Monitoring
In critical care settings, predictive analytics enhances patient monitoring by analyzing real-time data from multiple sources, such as ventilators, infusion pumps, and vital sign monitors. AI systems can predict conditions like sepsis or respiratory failure hours before they become clinically apparent, allowing clinicians to intervene early and improve patient outcomes.
- Behavioral Health and Mental Wellness
AI is also making strides in behavioral health by monitoring indicators of mental health conditions. For instance, algorithms can analyze speech patterns, social media activity, or smartphone usage to detect early signs of depression or anxiety. These insights enable mental health professionals to provide timely support, reducing the likelihood of crises.
Challenges and Ethical Considerations
While AI-driven predictive analytics offers immense potential, its implementation is not without challenges:
- Data Privacy and Security
Healthcare data is highly sensitive, and breaches can have serious consequences. Ensuring compliance with regulations like HIPAA and implementing robust cybersecurity measures are essential to protect patient privacy. - Bias in AI Models
AI systems can inherit biases from the data they are trained on, potentially leading to disparities in care. For example, if training datasets lack diversity, predictions may be less accurate for underrepresented populations. Addressing this requires careful dataset curation and continuous model evaluation. - Integration with Legacy Systems
Many healthcare institutions rely on legacy IT systems that are not easily compatible with modern AI tools. Achieving seamless integration and interoperability is a complex but necessary task. - Ethical Concerns in Decision-Making
AI-driven recommendations must balance automation with human oversight to maintain accountability. Clinicians should remain involved in critical decisions to ensure ethical and personalized care.
The Future of Predictive Analytics in Healthcare
The future of predictive analytics in healthcare is bright, with emerging trends pointing to even greater innovation:
- Genomic Medicine: AI can integrate genomic data with clinical insights to predict disease risks and recommend preventive strategies.
- AI-Driven Drug Development: Predictive models accelerate drug discovery by identifying promising compounds and forecasting their efficacy.
- Population Health Management: Public health initiatives can leverage AI to predict and prevent disease outbreaks, allocate resources, and design targeted interventions.
- Telemedicine and Virtual Care: Predictive analytics will enhance telehealth platforms, enabling clinicians to deliver data-driven care during virtual consultations.
Conclusion
AI-driven predictive analytics is transforming healthcare by enabling earlier detection, personalized care, cost reduction, and real-time patient monitoring. While challenges remain, addressing them with robust frameworks and ethical considerations will unlock the full potential of this technology. As healthcare systems increasingly embrace AI, predictive analytics will play a pivotal role in delivering high-quality, affordable, and accessible care to patients worldwide.
Written by: SAROWAR HOSSAIN
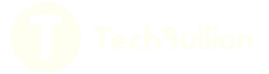