Share
Tweet
Share
Share
Introduction:
Artificial Intelligence (AI) is on the cusp of a major transformation, driven by the convergence of quantum computing and classical machine learning techniques. The hybrid quantum-classical approach represents a significant advancement, combining the strengths of both paradigms to tackle problems that are currently beyond the reach of classical systems alone. This research article delves into how these hybrid models can revolutionize various applications by enhancing computational efficiency and predictive power.
Quantum Computing Fundamentals:
Quantum computing is founded on the principles of quantum mechanics, which allows quantum bits (qubits) to exist in multiple states simultaneously—a phenomenon known as superposition. Unlike classical bits that are either 0 or 1, qubits can be both at once, leading to a vast increase in computational power. Additionally, quantum entanglement enables qubits that are entangled to affect each other’s state instantaneously, regardless of distance. This section of the article explores these foundational concepts and their potential to accelerate computations in ways that classical computers cannot match.
Classical Machine Learning Models:
Classical machine learning models have been instrumental in driving progress across various domains by enabling systems to learn from data and make predictions. Techniques such as neural networks, decision trees, and support vector machines are well-established and have demonstrated robust performance in many scenarios. However, these models often face limitations in handling large-scale data and complex, high-dimensional problems. This part of the article reviews these classical methods, their applications, and their limitations, setting the stage for the introduction of quantum-enhanced approaches.
Integration of Quantum and Classical Models:
The hybrid quantum-classical model leverages quantum computing’s unique capabilities to complement and enhance classical machine learning techniques. One key area of integration is the use of quantum algorithms to optimize classical algorithms’ performance, such as quantum-enhanced optimization techniques that can improve training efficiency and accuracy. For instance, quantum algorithms like the Quantum Approximate Optimization Algorithm (QAOA) can be used to solve combinatorial problems more effectively than classical counterparts. The article details specific integration strategies, including quantum-inspired machine learning models and quantum-assisted data preprocessing, illustrating how these methods can address traditional machine learning challenges.
Case Studies and Applications:
To illustrate the practical benefits of hybrid models, the article presents several case studies across different fields:
- Drug Discovery: In pharmaceutical research, hybrid models can accelerate the identification of potential drug candidates by simulating molecular interactions with unprecedented precision. This application showcases how quantum-enhanced simulations can lead to faster and more accurate results compared to classical methods.
- Financial Forecasting: Quantum algorithms have the potential to revolutionize financial modeling by analyzing market trends and risk factors more efficiently. The article discusses how hybrid models can improve prediction accuracy and decision-making in finance.
- Climate Modeling: Addressing climate change requires analyzing vast amounts of environmental data. Hybrid models offer a way to process and interpret this data more effectively, providing better insights into climate patterns and potential mitigation strategies.
Future Directions:
Looking ahead, the integration of quantum computing with classical machine learning is poised to open new avenues for research and application. However, there are challenges to overcome, such as developing scalable quantum algorithms and addressing issues related to quantum hardware limitations. The article highlights ongoing research efforts and potential breakthroughs that could address these challenges. Additionally, it discusses the broader implications of hybrid models for AI, including their impact on industry practices and future technological advancements.
Conclusion:
The hybrid quantum-classical approach represents a promising frontier in AI research, offering the potential to overcome limitations of classical systems and achieve breakthroughs in various domains. By combining quantum computing’s computational power with classical machine learning’s established techniques, researchers and practitioners can push the boundaries of what is possible, paving the way for a new era of AI innovation.
For full Article is available here: https://thesciencebrigade.com/jst/article/view/67/125
Read More From Techbullion
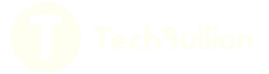