Share
Tweet
Share
Share
Cutting-edge integration patterns in unified AI and cloud platforms are systematically explored by Sushil Prabhu Prabhakaran, who offers a comprehensive review of process automation technologies. His innovative work bridges the gap between traditional AI deployment and the scalable, efficient solutions demanded by modern industries.
The Core of Unified Platforms
Unified AI and cloud platforms represent a paradigm shift in enterprise computing. By converging AI capabilities with the scalability of cloud infrastructure, these platforms redefine how organizations deploy and manage AI-driven processes. The architectural frameworks underpinning these systems integrate MLOps pipelines, workflow orchestration engines, and advanced data processing mechanisms. Together, these components enable dynamic resource allocation, automated governance, and seamless lifecycle management of machine learning models.
A foundational innovation within these platforms is their ability to support real-time data ingestion and transformation. This capability ensures that businesses can make instantaneous, data-driven decisions. Additionally, the incorporation of federated learning empowers distributed systems to train models without compromising data privacy.
Redefining Real-Time Architectures
Real-time processing architectures have been reimagined to address the growing need for instantaneous decision-making. These systems leverage in-memory computing and advanced streaming technologies to process data in milliseconds. By implementing fault-tolerant mechanisms and dynamic load balancing, unified platforms maintain consistent performance even under fluctuating workloads.
One groundbreaking feature is the edge-to-cloud communication model. This innovation optimizes interactions between edge devices and central cloud infrastructure, ensuring synchronized operations across distributed environments. The result is a robust system that excels in environments requiring high-speed, high-volume data processing.
Interoperability Across Platforms
Cross-platform interoperability is a cornerstone of unified AI systems. By employing standardized interfaces and hybrid deployment strategies, these platforms enable seamless integration across diverse computing environments. Multi-cloud frameworks further enhance this capability by facilitating secure and efficient operations across multiple providers.
Such interoperability not only reduces vendor lock-in but also ensures that businesses can scale operations based on performance demands and cost considerations. Automated orchestration mechanisms dynamically allocate resources, ensuring optimal utilization and consistent performance across all systems.
Governance and Explainability
With increasing adoption of AI, ensuring compliance and explainability has become paramount. Unified platforms address these challenges through robust governance frameworks that incorporate automated compliance checks and ethical oversight. These frameworks ensure adherence to regulatory standards and foster trust in AI systems.
Explainability mechanisms, such as integrated gradients and model-agnostic explanations, provide insights into AI decision-making processes. These tools cater to both technical experts and non-technical stakeholders, ensuring that AI operations remain transparent and accessible.
Emerging Trends in AI Platforms
The future of unified AI platforms lies in their ability to integrate with emerging technologies. Innovations such as extended reality (XR) and IoT are poised to redefine human-AI interactions. Edge computing is evolving to support more sophisticated processing capabilities, further blurring the lines between on-premise and cloud environments.
Addressing scalability challenges is another critical area. Advanced orchestration mechanisms and zero-trust security architectures are being developed to ensure reliable and secure operations. Sustainability initiatives, including green AI practices, are also gaining traction, highlighting the industry’s commitment to environmental responsibility.
Strategic Recommendations for the Future
To stay ahead in the rapidly evolving AI landscape, organizations must prioritize specific strategies for unified platform implementation. Focusing on developing standardized interfaces will ensure seamless interoperability across hybrid and multi-cloud environments. Additionally, investment in AI governance mechanisms will enhance transparency and ethical compliance.
Future research should explore sustainable AI practices, such as energy-efficient computing and optimized resource management. These initiatives will align technological advancement with environmental goals, creating a balance between innovation and sustainability. By incorporating advanced explainability tools, businesses can further bridge the gap between technical systems and user comprehension, fostering trust in AI-driven solutions.
In conclusion, Sushil Prabhu Prabhakaran’s work underscores the transformative potential of unified AI and cloud platforms. By bridging the gap between innovation and practical implementation, these systems offer scalable, efficient solutions to modern challenges. As industries continue to evolve, the insights from this research will serve as a foundation for future advancements in AI integration and automation. Unified AI platforms promise not only to redefine enterprise computing but also to set new benchmarks for scalability, transparency, and sustainability.
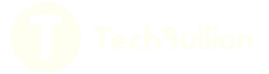
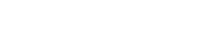